2016 International Conference on Intelligent
Computation
August 2-5,2016
Lanzhou,China
(
http://www.ic-icc.cn/2016/index.htm
)
The ICIC 2016 Program Committee is inviting proposals for tutorials to be held during the conference ( http://www.ic-icc.cn/2016/index.htm ), taking place on August 2-5, 2016, in Lanzhou, China.
Each tutorial session proposal should address hot and advanced Intelligent Computing topics. Tutorial session proposals should include title, outline, expected enrollment, and presenter biography with e-mail/website address.
Proposals for Tutorial sessions should be submitted in ELECTRONIC FORMAT to Tutorial Chairs:
Laurent Heutte
Université de Rouen, France
Laurent.Heutte@univ-rouen.fr
Abir Hussain
Liverpool John Moores University, UK
A.Hussain@ljmu.ac.uk
Important Deadlines:
Tutorial session proposal deadline:
May 1, 2016
Decision notification: May 20, 2016
Deep Learning and Computational Models of Human Audio-Visual Pathways
Soo-Young Lee
Professor, School of
Electrical Engineering
Director, Brain Science
Research Center
Korea Advanced Institute of
Science and Technology
Daejeon, 34301,
Republic of Korea
sy-lee@kaist.ac.kr
http://cnsl.kaist.ac.kr/,
http://bsrc.kaist.ac.kr/new/english/main.htm
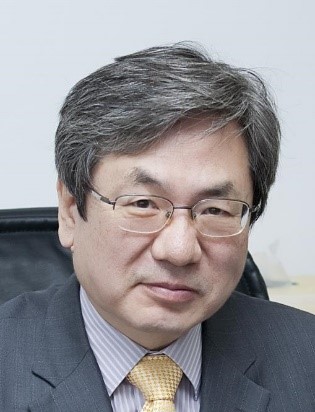
Abstract: Recently deep learning had attracted a lot of attention from both academic and industrial communities for speech, image, and video recognition tasks. This course will bring the connection between deep neural networks and cognitive computational models of human audio-visual information processing. The cognitive scientific facts on information processing mechanism in human audio and visual pathways will be first introduced, and the computational models in the form of deep neural architecture and learning algorithms will be studied for the hierarchical feature extraction, stereo/binaural spatial information processing, selective attention, and audio-visual integration. Graduate students and researchers who are interested in understanding the essential theory and practical basics on deep learning.
Human audio-visual processing starts from feature extraction in hierarchical manner from local to global features at cochlear and retina, respectively. Although there exists controversy on the merit of unsupervised initialization, it is believed to be logical to start from genetically-coded feature extractors and latter go through learning process for more discriminant feature extractors. Especially for small and medium size training data, unsupervised initialization of feature extractors, mimicking learning process in million years of human history, is advantageous. The popular unsupervised learning algorithms for the feature extraction include PCA (Principal Component Analysis), NMF (Non-negative Matrix Factorization), RBM (Restricted Boltzmann Machine), ICA (Independent Component Analysis), and recently AE (Auto-Encoder). For audio processing model, similar to features extracted at basilar membrane at cochlear, we shows that time-frequency-constrained local features may be learned by ICA may result in better initialization, especially for convolutional neural networks. These unsupervised learning algorithms are the basic component of hierarchical feature extractors at the early layers of deep CNNs (Convolutional Neural Networks). At the latter layers of deep CNNs these features are combined to make classification decision with a multi-layer Perceptron, which is learned by error back-propagation learning algorithm. These deep network architecture, with deterministic or stochastic neurons, and also popular ReLU (Rectified Linear Unit) nonlinearity are found at human audio-visual pathways. Also, the recently-resurgent gate-based attentive networks has its biological plausibility from the top-down synaptic connections. Actually, there are much more top-down connections than bottom-up connections in audio-visual pathways. To combine the audio and visual information the biological networks may utilize so-called “late integration” approach such as Committee Machines. However, to explain McGurk effect more clearly for incongruent audio and visual signals, the gate-based top-down attention model may be applied to audio-visual integration.
Bio-Sketch: Soo-Young Lee received B.S., M.S., and Ph.D. degrees from Seoul National University in 1975, Korea Advanced Institute of Science in 1977, and Polytechnic Institute of New York in 1984, respectively. From 1977 to 1980 he worked for the Taihan Engineering Co., Seoul, Korea. From 1982 to 1985 he also worked for General Physics Corporation at Columbia, MD, USA. In early 1986 he joined the Department of Electrical Engineering, Korea Advanced Institute of Science and Technology, as an Assistant Professor and now is a Full Professor at the Department/School of Electrical Engineering. He was also the founding Chairman of the Department of Bio & Brain Engineering at KAIST. From June 2008 to June 2009 he also worked for Mathematical Neuroscience Laboratory at RIKEN Brain science Institute for his sabbatical leave.
In 1997 he established Brain Science Research Center, which is the main research organization for the Korean Brain Neuroinformatics Research Program, of which two goals are to understand brain mechanism and also to develop intelligent machine based on the algorithm. The research program is one of the Korean Brain Research Promotion Initiatives sponsored by Korean Government from 1998 to 2008, and about 35 Ph.D. researchers had joined the research program from many Korean universities.
He is a Past-President of Asia-Pacific Neural Network Assembly (APNNA), and currently President-Elect of its decent, the newly-founded Asia-Pacific Neural Network Society (APNNS). He has contributed to International Conference on Neural Information Processing as Conference Chair (2000), Conference Vice Co-Chair (2003), and Program Co-Chair (1994, 2002). He is on Editorial Boards for Neural Processing Letters and Cognitive Neurodynamics journals. He received Leadership Award and Presidential Award from International Neural Network Society in 1994 and 2001, respectively, and APPNA Service Award and APNNA Outstanding Achievement Award in 2004 and 2009, respectively. From SPIE he also received Biomedical Wellness Award and ICA Unsupervised Learning Pioneer Award in 2008 and 2010, respectively.
His research interests have resided in Artificial Brain, alias Artificial Cognitive Systems, the human-like intelligent systems/robots based on biological information processing mechanism in our brain. He has worked on computational models of the auditory and visual pathways, unsupervised and supervised learning architecture and algorithms, active learning, situation awareness from environmental sound, and top-down selective attention. From late 2000s he had extended his research into higher cognitive functions such as NLP (natural language processing) and understanding human internal states. For the latter he had started from cognitive neuroscience experiments with multimodal data including fMRI, EEG, and eye movements, and is pioneering a new research area to identify human internal states, such as memory, agreement to others, and trustworthiness of others, with EEG and eye movements. His research scope covers cognitive experiments, mathematical models, and real-world applications.
References
- K. Fukushima, S. Miyake, and T. Ito, “Neocognitron: A neural network model for a mechanism of visual pattern recognition,” IEEE Transactions on Systems, Man, and Cybernetics Volume: SMC-13, Issue: 5, pp. 826 �C 834, 1983.
- Y. LeCun, Y. Bengio, & G. Hinton, “Deep Learning”, Nature, 521, 436�C444, 2015.
- J. Lee and S.Y. Lee, “Deep learning of speech features for improved phonetic recognition,” Twelfth Annual Conference of the International Speech (Interspeech2011), 2011.
- J.H. Lee, H.Y. Jung, T.W. Lee, and S.Y. Lee, " Speech feature extraction using independent component analysis", IEEE International Conference on Acoustics, Speech, and Signal Processing (ICASSP 2000), 2000.
- T. Kim and S.Y. Lee, "Learning Self-organized Topology-preserving Complex Speech Features at Primary Auditory Cortex", Neurocomputing, Vol. 65-66, pp. 793-800, 2005. 06.
- M.J. Lee and S.Y. Lee, "Unsupervised Extraction of Multi-Frame Features for Lip-Reading", Neural Information Processing-Letters and Reviews, Vol. 10, No. 4-6, pp. 97-104, 2006. 06.
- M.J. Lee, A.S. Lee, D.K. Lee, and S.Y. Lee, “Video Representation with Dynamic Features from Multi-Frame Frame-Difference Images,” IEEE Workshop on Motion and Video Computing (WMVC'07), 2007.
- C.S. Dhir and S.Y. Lee, “Discriminant Independent Component Analysis,” IEEE Trans. Neural Networks, Vol.22, No.6, pp. 845�C857, 2011.06.
- S.Y. Lee, H.A. Song, S. Amari, “A new discriminant NMF algorithm and its application to the extraction of subtle emotional differences in speech,” Cognitive Neurodynamics, Vol. 6, pp. 525-535, 2012.
- H.A. Song, B.K. Kim, T.L. Xuan, and S.Y. Lee, “Hierarchical feature extraction by multi-layer non-negative matrix factorization network for classification task,” Neurocomputing, Volume 165, Pages 63�C74, October 2015.
- J.I. Lim and S.Y. Lee, "Unified Training of Feature Extractor and HMM Classifier for Speech Recognition”, IEEE Signal Processing Letters, Vol. 19, Issue 2, pp. 111-114, 2012.
- B.T. Kim and S.Y. Lee, "Sequential Recognition of Superimposed Patterns with Top-Down Selective Attention", Neurocomputing, Vol. 58-60, pp. 633-640, 2004. 06.
- S.Y. Jeong and S.Y. Lee, "Adaptive Learning Algorithm to Incorporate Additional Functional Constraints into Neural Networks", Neurocomputing, Vol. 35, pp. 73-90, 2000. 11.
Table of Contents
- Introduction: Connecting Audio-Visual Human
Perception and Deep Learning
- Current Status of Deep Learning
- Physiological Basics of Human Auditory and Visual Systems
- Biological Plausibility of Deep Learning
- Feature Extraction
- Feature Extraction in Human Visual Pathway
- Feature Extraction in Human Auditory Pathway
- Unsupervised Feature Learning Algorithms (PCA, RBM, NMF, AE, and ICA)
- Hierarchical Feature Learning Algorithms
- Classification
- Perception in Human Audio-Visual Pathway
- Supervised Discriminant Learning Algorithm of Multi-layer Perceptron (EBP)
- Fine-Tuning of both Feature Extractors and Classifier
- Audio-Visual Integration
- Audio-Visual Perception in Human Audio-Visual Pathways
- Committee Machines for Multi-modal Integration
- Top-Down Attention for Multi-modal Integration
- Important Issues
- Deterministic (Sigmoid, ReLU, etc.) vs. Stochastic Neurons
- Summation vs. Multiplication (Gated) Neurons
- Unsupervised vs. Random Initialization for Learning Convergence
- Regularization (Dropout, Saturation/Low-Sensitivity, etc.)
- Parallelization
- Future Directions